2025-02-07
Oncology: When AI Takes Control…
Oncology
#Oncology #ArtificialIntelligence #Cancer #NewTherapy
The integration of artificial intelligence (AI) in oncology marks a revolution in the management of cancer patients. Thanks to its advanced analytical capabilities, AI efficiently processes narrative data, which constitute more than 80% of the information contained in electronic medical records. These data, often difficult to exploit manually, include radiology reports, clinical observations, and complex therapeutic protocols. By structuring these data, AI facilitates clinical decision-making by organizing and analyzing medical information. It helps personalize treatments and detect predictive trends invisible to traditional methods.
However, despite these advances, the widespread adoption of AI in oncology remains a challenge. Algorithmic biases are a major concern, particularly when they stem from unrepresentative or incomplete training data.
Additionally, clinical validation of these tools remains insufficient. Questions persist regarding their reliability and effectiveness in real-world environments. Finally, the interpretability of AI models is a significant obstacle. Healthcare professionals hesitate to adopt systems whose decision-making processes remain opaque, even when they produce impressive results.
This study evaluates the effectiveness of natural language processing (NLP) models in improving the interpretation and analysis of narrative medical data in oncology. It examines their ability to predict prognoses, recommend personalized treatments, and match patients to clinical trials. In parallel, additional approaches such as federated learning were explored to ensure data confidentiality and improve model generalization. These tools were tested under real clinical conditions to identify their limitations and areas for improvement.
This study demonstrates that NLP models exhibit high accuracy, sometimes surpassing human performance in predicting cancer progression and recommending treatments. However, their clinical application remains limited by challenges such as the lack of data standardization and the management of errors in complex cases. These advances confirm AI’s potential in oncology, while also highlighting the need for further improvements to enable broader adoption.
This study aimed to evaluate the effectiveness of AI models in oncology, examining their ability to interpret medical data, predict cancer progression, and recommend tailored treatments. It highlights both their potential and current limitations.
The results indicate that AI can surpass human expertise in certain tasks, such as prognosis prediction and patient matching for clinical trials.
However, its clinical integration faces multiple challenges. Biases in training data can distort predictions, and the complexity of models hinders adoption. Moreover, the lack of standardization and the opacity of algorithms pose risks, requiring rigorous validation to ensure their safety and reliability. Finally, the environmental footprint of complex models and the need to build trust among clinicians slow down adoption.
In the future, multicenter evaluations and ethical integration will be essential to ensure the reliability of these technologies. Stronger regulations, combined with more explainable and eco-friendly models, could make AI a central pillar of oncology, offering more precise, personalized, and accessible treatments.
The integration of artificial intelligence (AI) in oncology marks a revolution in the management of cancer patients. Thanks to its advanced analytical capabilities, AI efficiently processes narrative data, which constitute more than 80% of the information contained in electronic medical records. These data, often difficult to exploit manually, include radiology reports, clinical observations, and complex therapeutic protocols. By structuring these data, AI facilitates clinical decision-making by organizing and analyzing medical information. It helps personalize treatments and detect predictive trends invisible to traditional methods.
However, despite these advances, the widespread adoption of AI in oncology remains a challenge. Algorithmic biases are a major concern, particularly when they stem from unrepresentative or incomplete training data.
Additionally, clinical validation of these tools remains insufficient. Questions persist regarding their reliability and effectiveness in real-world environments. Finally, the interpretability of AI models is a significant obstacle. Healthcare professionals hesitate to adopt systems whose decision-making processes remain opaque, even when they produce impressive results.
This study evaluates the effectiveness of natural language processing (NLP) models in improving the interpretation and analysis of narrative medical data in oncology. It examines their ability to predict prognoses, recommend personalized treatments, and match patients to clinical trials. In parallel, additional approaches such as federated learning were explored to ensure data confidentiality and improve model generalization. These tools were tested under real clinical conditions to identify their limitations and areas for improvement.
Read next: AI-based pathology predicts origins for cancers of unknown primary
Can AI Truly Revolutionize Oncology?
To validate the effectiveness of these NLP models, they were trained using supervised and transfer learning. Their efficiency was measured by analyzing the following outcome variables: accuracy, patient survival, and the relevance of therapeutic recommendations. The integration of federated learning allowed institutions to collaborate without directly sharing their data, ensuring confidentiality while improving model generalization.This study demonstrates that NLP models exhibit high accuracy, sometimes surpassing human performance in predicting cancer progression and recommending treatments. However, their clinical application remains limited by challenges such as the lack of data standardization and the management of errors in complex cases. These advances confirm AI’s potential in oncology, while also highlighting the need for further improvements to enable broader adoption.
Read next: L’IA dans le cancer du poumon non à petites cellules
AI and Cancer: A Revolution in Progress or an Illusion?
Cancer remains a leading cause of mortality worldwide, necessitating continuous advancements to optimize diagnoses and treatments. In this context, AI emerges as a powerful tool to enhance oncology care, particularly through data analysis and personalized treatments.This study aimed to evaluate the effectiveness of AI models in oncology, examining their ability to interpret medical data, predict cancer progression, and recommend tailored treatments. It highlights both their potential and current limitations.
The results indicate that AI can surpass human expertise in certain tasks, such as prognosis prediction and patient matching for clinical trials.
However, its clinical integration faces multiple challenges. Biases in training data can distort predictions, and the complexity of models hinders adoption. Moreover, the lack of standardization and the opacity of algorithms pose risks, requiring rigorous validation to ensure their safety and reliability. Finally, the environmental footprint of complex models and the need to build trust among clinicians slow down adoption.
In the future, multicenter evaluations and ethical integration will be essential to ensure the reliability of these technologies. Stronger regulations, combined with more explainable and eco-friendly models, could make AI a central pillar of oncology, offering more precise, personalized, and accessible treatments.
Read next: L’IA dans l’évaluation de l’adénocarcinome pulmonaire
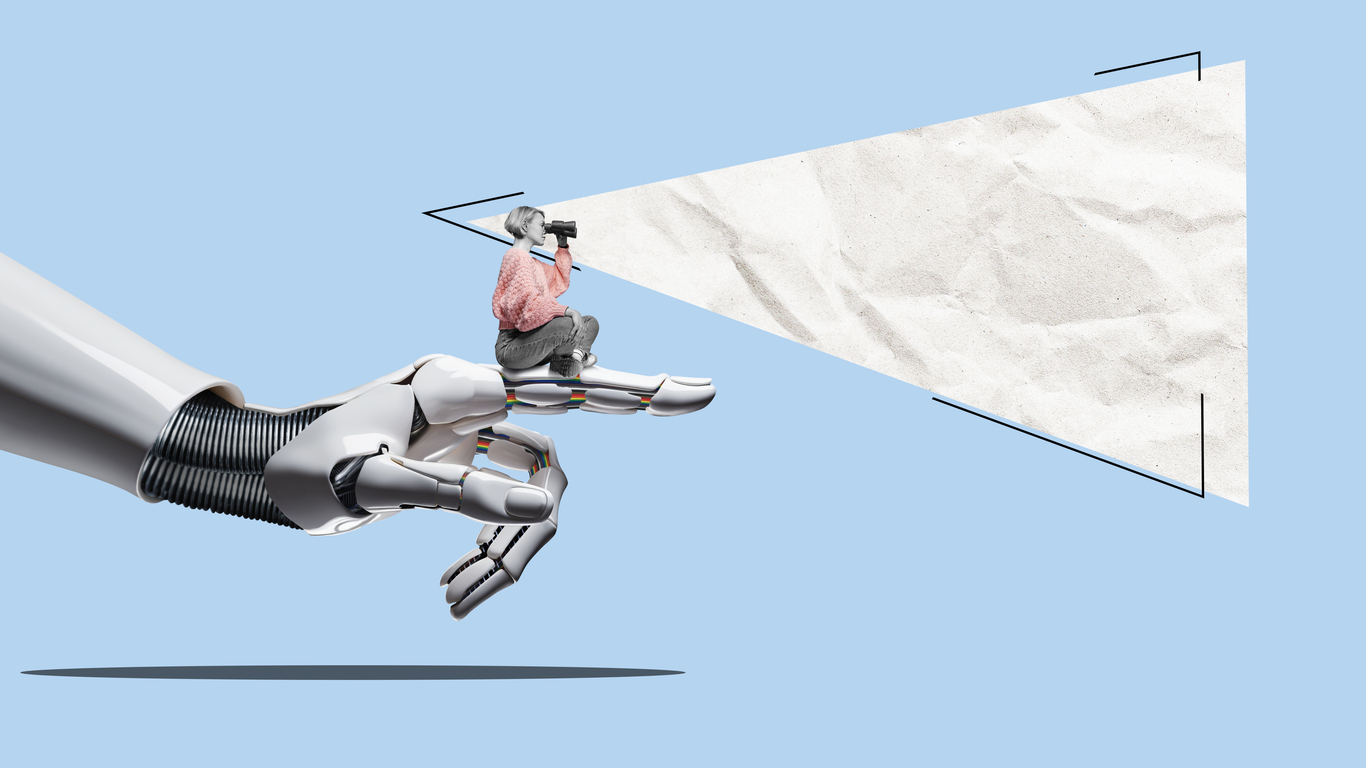
Last press reviews
Oncology: When AI Takes Control…
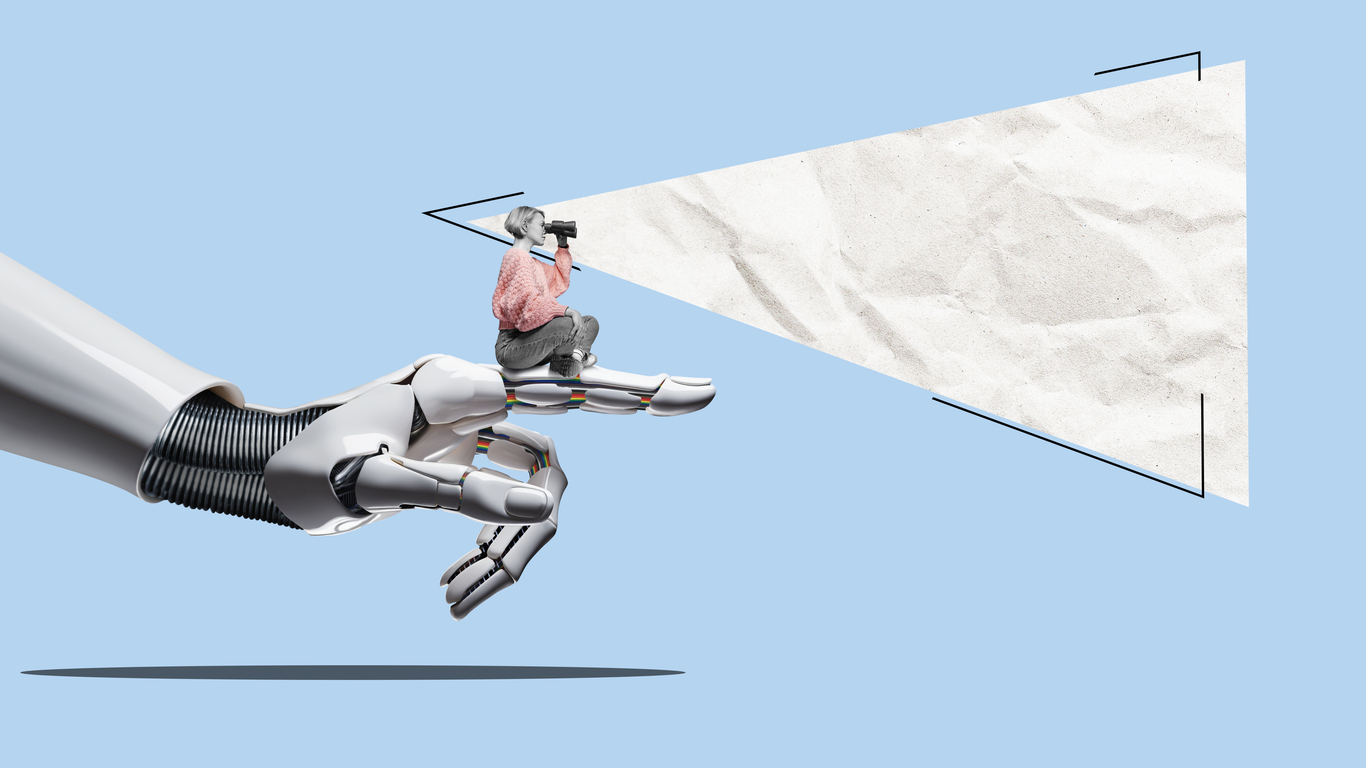
#Oncology #ArtificialIntelligence #Cancer #NewTherapy...
Radium-223 & Enzalutamide: A Powerful Duo Against mCRPC!
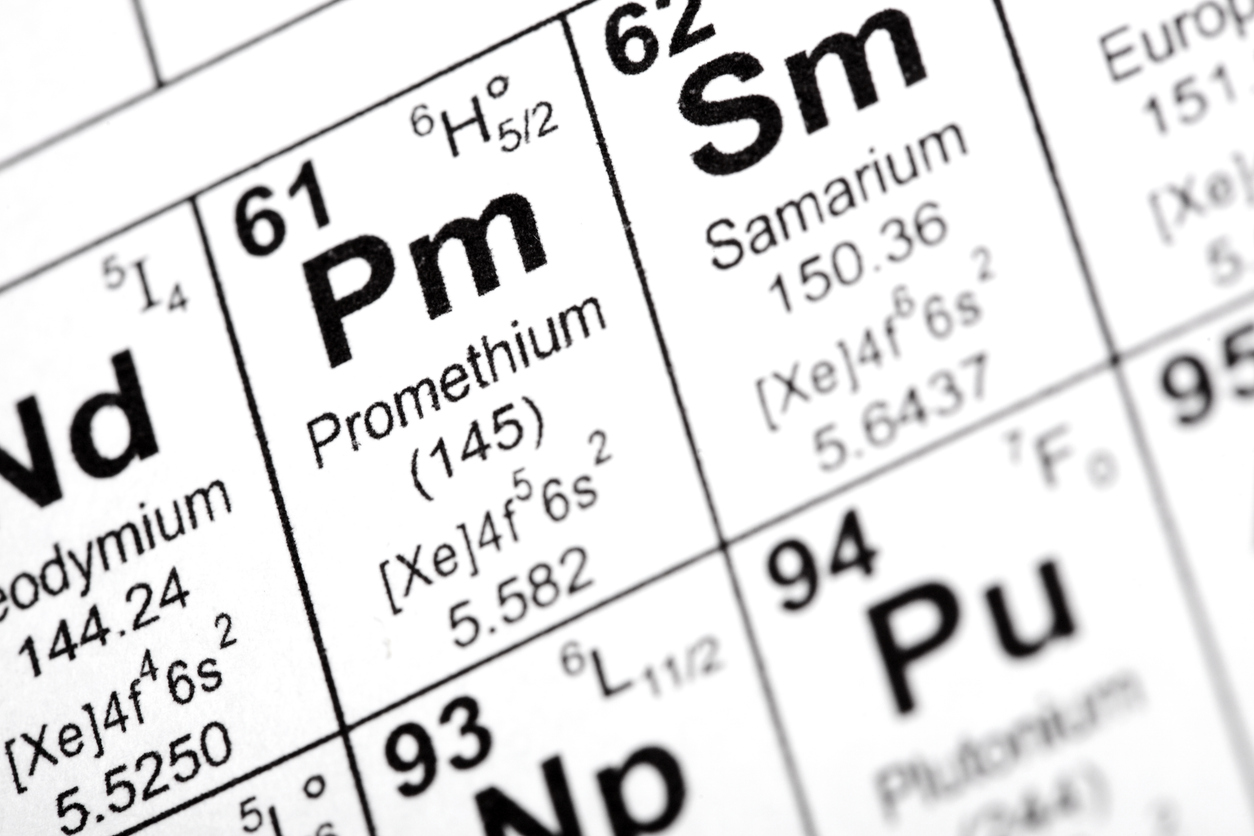
#Oncology #ProstateCancer #mCRPC #Radium223 #Enz...
NIAGARA: A Wave of Hope for Invasive Bladder Cancer
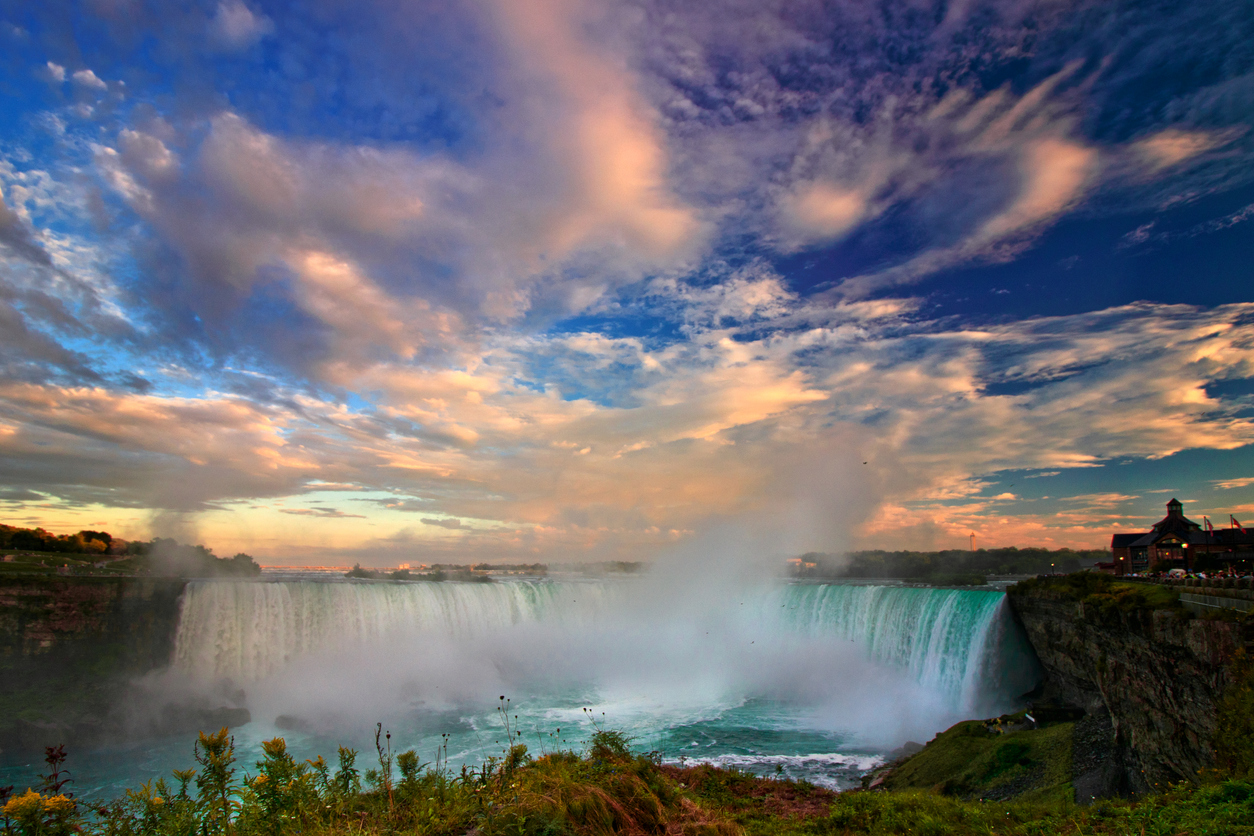
#Oncology #MIBC #Immunotherapy #Chemotherapy #NI...